Companies and researchers in many fields, including animal science, are eager to integrate artificial intelligence (AI) into every product and service. Various tech experts claim that AI will revolutionize all aspects of animal production and replace human jobs. They suggest that animal agriculture will soon be performed on a computer with very little human input. However, this is far from reality. In this post, we will explore why AI is currently more of a speculative bubble, primarily generating speculative value for certain startups, companies, and research programs, rather than a tool that effectively addresses pressing issues in animal nutrition. We will also explain why, as an animal scientist, producer, or anyone involved in livestock production, you don’t need to worry about these technologies taking your job.
AI is not intelligent
Artificial intelligence is a branch of computer science that has been studied for decades and has developed incredible tools that are used in our daily lives. However, the purpose of this post is not to discuss AI as a scientific field in depth, but rather to examine AI as a marketing term used by many companies and scientists to promote a “disruptive” technology that claims to solve the most pressing problems in animal agriculture due to its tremendous computational power and intelligence.
Although these computer systems are called intelligent, they are not. These systems lack the ability to solve problems that we have not yet solved, such as many that exist in current biology, and thus they are of very limited scope in the field of animal sciences. Let’s look at the capabilities and limitations of AI, analyzing its strengths and weaknesses using various examples. This exploration is expected to shed light on both the potential applications and limitations of AI systems in the field of animal sciences.
What a parrot can do?
ChatGPT (Chat Generative Pre-trained Transformer) is a chatbot that responds coherently to natural language questions and is commonly regarded as intelligent. This system was developed by feeding it a large, structured collection of symbols, such as text, images, or video frames, and it outputs a similarly structured collection of symbols. ChatGPT uses probability to determine which word, pixel, or video frame is most likely to appear next. Essentially, it is an imitation system.
If we feed every piece of text on the internet into a generative AI like ChatGPT, it can produce text that resembles any other piece of text on the internet. A system like this is very useful if we need to generate text, audio, or video, but it is not intelligent at all. Nevertheless, due to some human biases, we tend to believe these imitation models are intelligent.
Bender et al. (2021) in their paper “On the Dangers of Stochastic Parrots: Can Language Models Be Too Big?” mentioned that “the tendency of human interlocutors to impute meaning where there is none can mislead both Natural Language Processing researchers and the general public into taking synthetic text as meaningful.” This means that people often attribute genuine meaning to text generated by AI, even when the AI is simply producing text based on patterns in data without any understanding. This tendency can lead both researchers and the general public to believe that systems like ChatGPT are truly intelligent, when in fact they are simply sophisticated mimics of human language or, as Bender et al. describe it, a stochastic parrot.
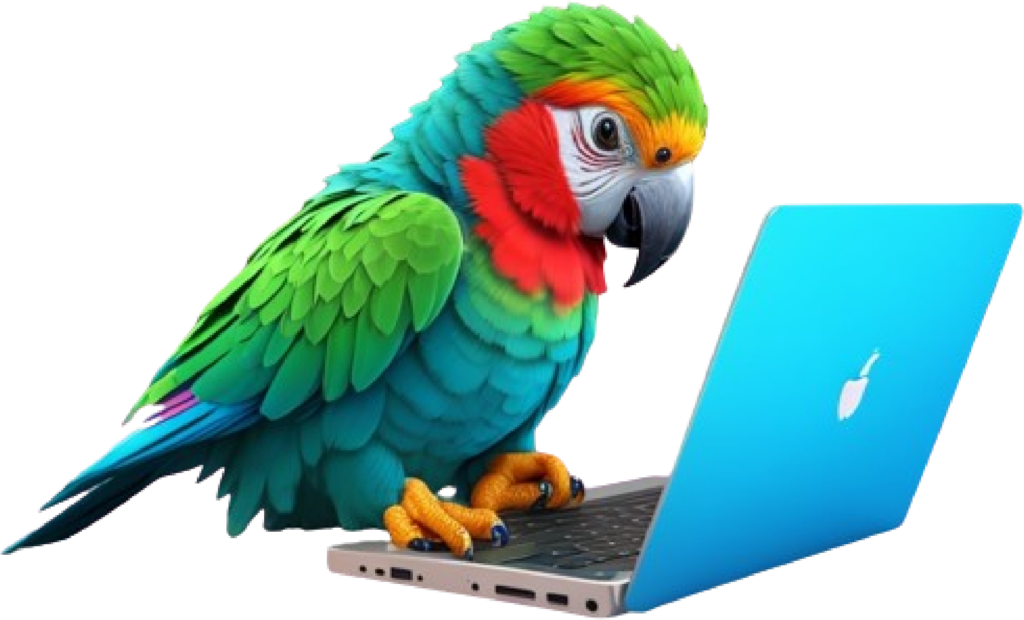
The unrecognized workforce behind AI
Although AI systems are considered computational systems, they rely more on humans than on computers and frequently depend on underpaid individuals to make them operational. For example, a Time Magazine investigation revealed that OpenAI, the company that developed ChatGPT, employed tens of thousands of Kenyan workers, paying them between $1.32 to $2 per hour to filter out violent, sexually explicit, or disturbing content from being used in the training data. In addition, AI systems like ChatGPT utilize the work of millions of humans without compensating them or respecting copyright laws. Generative AI systems can imitate any kind of structured collection of symbols or pixels if they are provided with an enormous amount of training data to copy from. However, the original work was developed by the intellect of millions of humans who were never compensated for their contributions to improving the model’s accuracy. Without the original work of millions of humans and the extensive data cleaning process involving significant manpower, these AI systems would be useless.
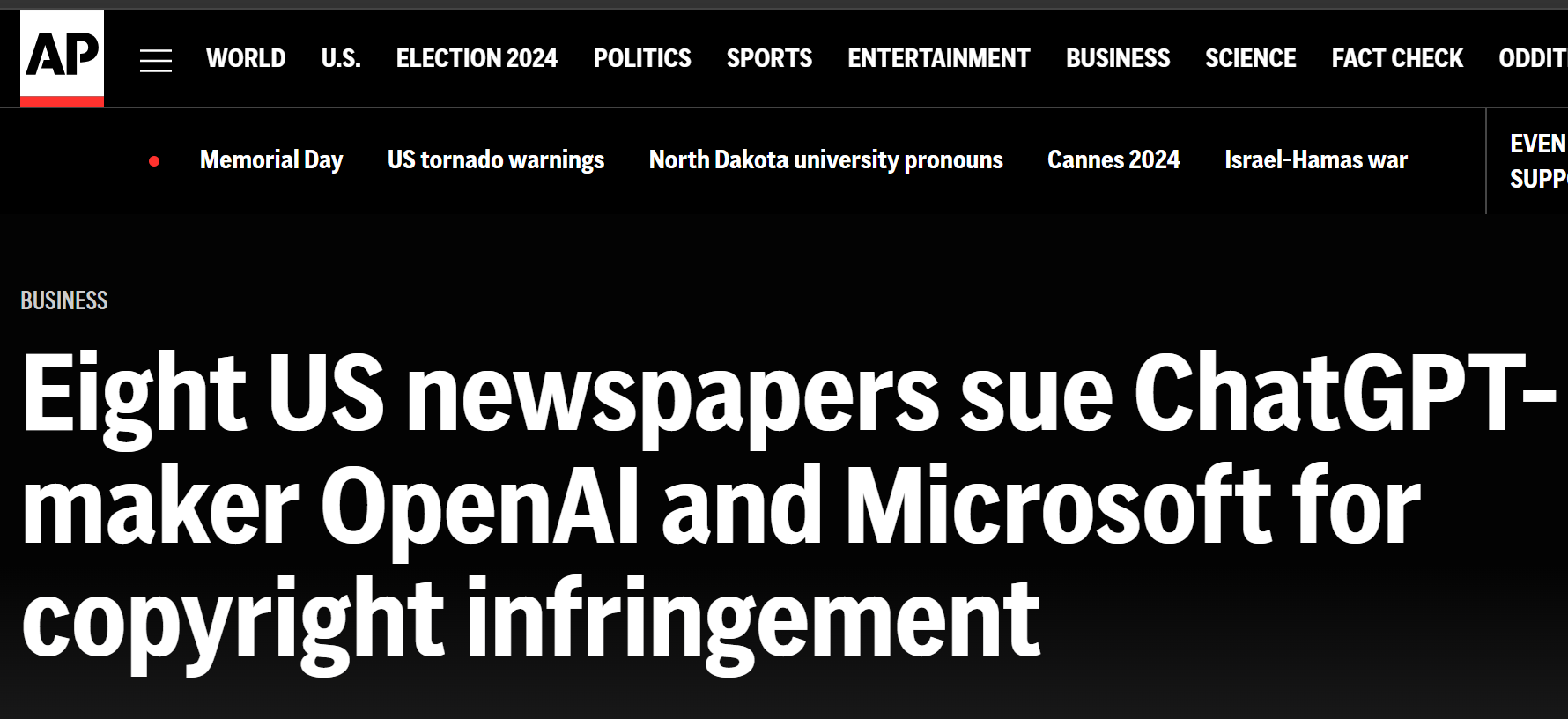
Multiple lawsuits are in place against OpenAI for using copyrighted data to train their model.
Thus, generative AI systems like ChatGPT rely on the intelligence of millions of people and the work of tens of thousands of individuals to function effectively. This context is crucial for understanding why similar AI systems won’t exist, at least in the short term, in animal production. Companies in this field lack the vast amount of data and manpower needed to develop such systems. However, this is not the main reason why sophisticated AI systems are currently science fiction in the field of Animal Sciences. The main reason lies in the nature of the questions we are asking these AI systems to answer, and the lack of well-defined knowledge structures needed to address those questions.
Knowledge structures
Generative AI systems like ChatGPT predict what words or groups of words are most likely to appear next based on the input they receive. This is indeed a conceptually straightforward task: predicting an output based on the Knowledge structures (an arrangement of knowledge elements or systematic information) we have provided. As humans, we have implicitly given ChatGPT-like systems the knowledge of what words come next in the texts we have been writing for thousands of years. In essence, we have provided the AI system with the answer to the problem by supplying it with the historical data it needs to learn and generate coherent text. The AI system, by itself, cannot generate any meaningful output without clear and defined knowledge structures. This consideration is important, as in many applications in the Animal Sciences, these AI technologies are used without any clear predefined knowledge structure, expecting the system to provide it for us. In that scenario, AI models simply don’t work as these models are not truly intelligent.
To perform decently, any AI model needs to be trained with well-defined knowledge structures, such as structured text or images. For example, in the field of medicine, if we provide an object recognition model with photos of tumors, these systems can identify them in CT scan images even more accurately than humans. Similarly, in the field of plant science, we can input thousands of images of various plant diseases into an object recognition model, and the model can then determine which disease is most likely depicted in a given photo. However, what these models cannot do is define what constitutes a tumor or a disease; they rely on pre-existing human-defined text, photos, categories and/or labels to function (i.e., knowledge structures).
Descriptive vs predictive AI
When attempting to apply AI models to the realm of animal production, we must consider that without a well-defined knowledge structure for addressing our problem, AI cannot solve it for us. For example, in the field of Swine production, a significant issue is the high mortality rate of over 30% of animals between the peripartum period and market, with causes not fully understood. Therefore, without a comprehensive understanding of the causes and a solid knowledge structure outlining how to tackle the problem, it’s impossible to train an AI model to accurately identify them. Nevertheless, we can still use sophisticated machine learning techniques that can be considered as AI. However, in this context, these models serve more of a descriptive role than a predictive one.
For example, in a study conducted by Rahman et al. (2023) using machine learning models, it was found that among the main factors contributing to pre-wean mortality in pigs were litter size, piglet birth weight, gestation length, and parity. These variables cannot be easily altered or modified within current production systems, resulting in a lack of predictive and prescriptive power in these AI technologies. In this context, we may first need to investigate more fundamental questions, such as what factors affect fetal development in large litters or which factors influence gestation length. This way, we can effectively use AI technologies as predictive tools that aid in decision making.
By addressing fundamental questions first, we can create the knowledge structure necessary for AI models to mimic. It is important to emphasize that AI models need knowledge structures as input, not just raw data. The data inputs for these models must be structured and clean. This is similar to the work done by tens of thousands of Kenyan workers for ChatGPT, and it is the kind of foundational work that animal scientists need to create to develop effective AI models.
Reading text vs reading animals
There are additional complexities to consider when utilizing AI models in the realm of animal sciences. Models like ChatGPT are designed primarily for predicting within entirely human-made systems, such as language. However, in the field of animal sciences, our goal is to forecast the behavior of biological systems that have evolved over millions of years, possess resilience, and of which we only understand a fraction. Consider the application of machine vision to study animal behavior. Machine vision is a computational system that looks for patterns in video or image footage to identify objects or events. In this context, we have used machine vision for more than a decade to identify animal behavior such as lying, walking, scratching, eating, etc. With the current technology, identifying animal behavior has become relatively straightforward, supported by thousands of practical examples available online. However, we have not yet determined the optimal behaviors for animals, such as the ideal duration for lying down, walking, scratching, or eating to achieve specific desired performance. This absence of established knowledge structures inhibits the model from predicting behaviors that could guide management strategies to enhance animal performance. Currently, we can accurately describe the pose or activity an animal is executing, but what does it mean? How do we account for animal “personality”? what is optimal behavior? If we haven’t deciphered animal behavior, AI won’t decipher it for us. Intelligence is not the output of an AI system; it is their input.
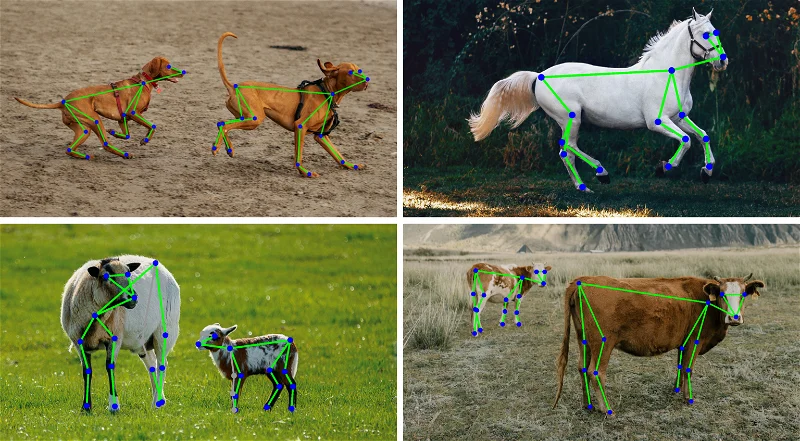
Image: Current technologies allow us to study animal pose and movement; however, we still need to define what is optimal behavior. Source: The Complete Guide to Animal Pose Estimation in 2023: Tools, Models, Tutorial; supervisely.com
Compared to other scientific disciplines, the application of AI systems in animal science faces more complex challenges due to the intricate nature of ecological systems. One notable challenge arises in utilizing machine vision to identify diseases or abnormal behaviors in animals. Animals have evolved over millions of years, developing adaptations aimed at evading predators. These adaptations include camouflage strategies and concealing vulnerabilities to avoid detection and targeting. Such evolutionary mechanisms present formidable obstacles for AI systems striving to accurately identify and interpret animal behaviors and health conditions. Some health conditions or diseases may not manifest obvious external signs in animals. For instance, oxidative stress or metabolic diseases may not exhibit visible symptoms, posing difficulties for AI systems to detect them. Additionally, in cases where animals display signs of lameness, arthritis might have developed weeks or months earlier, making it challenging to pinpoint the exact cause or onset of the issue. Furthermore, even when detected, it may already be too late to intervene effectively or economically. Therefore, the notion of a machine vision system detecting diseases in a barn appears to be akin to science fiction, especially for many diseases, as AI systems only mimic human intelligence, and we, as humans, haven’t fully solved this problem ourselves. This scenario parallels attempting to identify cancer or diabetes in an individual solely based on a photograph or daily video footage, a concept that may eventually become feasible but remains largely speculative at present.
Another important challenge that machine vision systems face in becoming practical is the need to perform at levels close to human intelligence, which is not an easy task. For example, if you have experience working in a sow barn, you probably know that when a sow has been lying down for some time and then starts to walk, it exhibits a movement pattern that resembles lameness. However, this lameness is transitory and does not represent a health challenge. For a trained animal caretaker, detecting this transitory lameness is easy. However, teaching a machine to recognize this scenario is very challenging because there are multiple cues that humans can intuitively understand or read, but these cues are difficult to explain or describe.
Because of the complexity of animal behavior, teaching a computer to recognize animal behavior, such as lameness, may require tens of thousands, if not more, hours of labeled video footage. This process involves substantial costs for collecting, storing, preprocessing, and cleaning data, as well as training and fine-tuning a model, transitioning it to production, and establishing a mechanism to tag lame animals in the field, among other challenges. Alternatively, it might be more practical to “read” animal behavior based on their eating or drinking habits, allowing for the development of less sophisticated but more predictive models. It’s important to note that the use of AI may often follow a technique-centric approach rather than a data-driven or problem-driven one, and although it may seem more advanced, in many cases, it represents the longest path to a solution.

Remember: Intelligence is not the output of an AI system; it is their input
Technique-centric AI
As highlighted earlier, the allure of perceived intelligence in imitation systems like generative AI in text prediction, often overshadows their actual predictive power in other fields, driving up their perceived value. This appeal attracts researchers and companies looking for innovative solutions, albeit at potentially higher costs compared to simpler methods. While sophisticated AI tools such as TensorFlow from Google, PyTorch by Meta, and VGG from Oxford University are freely available as open-source resources, the process of building knowledge structures (comprising data collection, cleaning, labeling, etc.) can demand substantial financial resources, sometimes amounting to millions of dollars, with outcomes remaining uncertain. Hence, it’s vital to weigh the expenses associated with machine vision and AI technologies and explore alternative methods that may provide effective solutions at lower costs. Emphasizing the importance of defining the problem and selecting the most suitable solution, it’s essential to recognize that AI is often the costliest option. Therefore, a thoughtful approach to AI development, considering both benefits and expenses, is crucial for successful implementation.
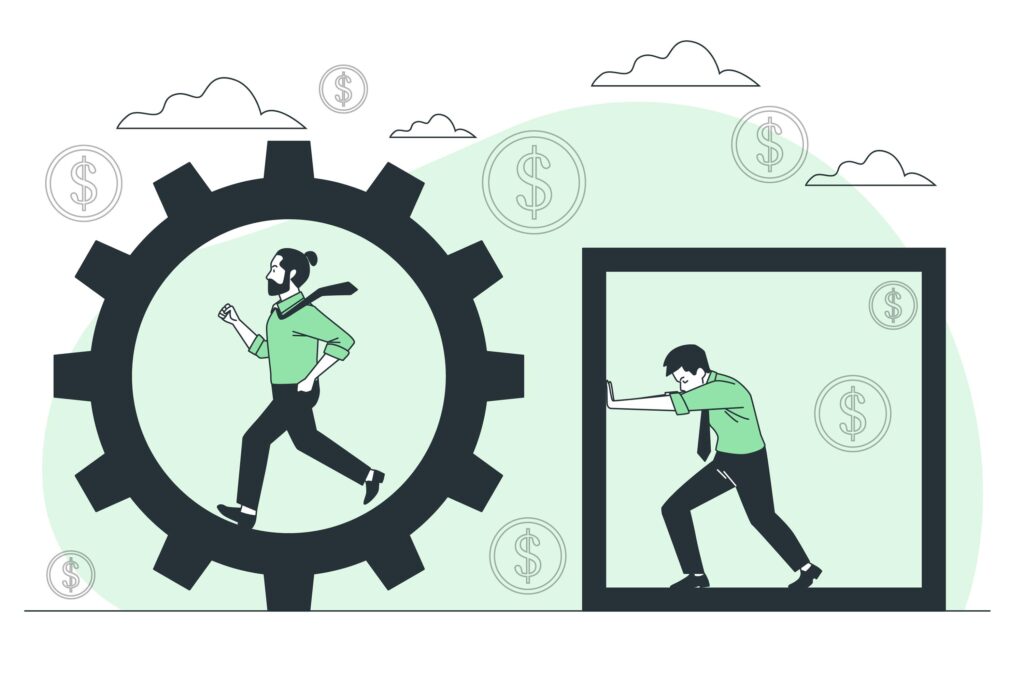
Garbage In, Garbage Out: Perpetuating Biases
AI’s seeming intelligence can perpetuate biases because its outputs are entirely reliant on inputs. These inputs, or knowledge structures, are shaped by developers’ beliefs about what constitutes sufficient data, labels, and assumptions. Consequently, AI systems merely mimic these biases and continue to use them in their predictions. This can lead to a mistaken belief that AI-generated solutions are optimal due to their computational power, when in reality, they are only as good as the assumptions and knowledge of the developer. The AI generates the best possible solution within its training data, but the scope of this data might not encompass the ideal solution for the problem at hand. Therefore, AI predictions cannot be considered optimal.
Consider monitoring animal nutritional requirements based on body condition. Management based on body condition scores assumes that optimal visual condition equates to optimal health. However, the body employs various strategies to maintain physical function and appearance even in suboptimal conditions. For example, when animals lack sufficient phosphorus in their diet, their bones adapt by slowing down the activity of osteoclasts, which break down bone, while osteoblasts, which generate new bone tissue, intensify their work. This adaptation reinforces bones, making them larger yet less dense, enabling them to withstand pressure despite lower phosphorus intake. However, this decreased bone density can lead to joint wear, potentially causing lameness. Nevertheless, not all animals develop lameness, but they can endure chronic inflammation processes, making them more susceptible to diseases. In such cases, video-based AI systems may not detect chronic inflammatory processes, leaving animals in suboptimal conditions despite showing “optimal body condition”. Consequently, an AI nutritional and health assessment system may prove ineffective, although the apparent intelligence of these systems could create a false impression of being optimal. In this case, to efficiently use AI models, we first need to answer the fundamental question: Is visual appearance the optimal way to assess health? If not, using machine vision may perpetuate suboptimal practices.
Don’t fall into the AI marketing trap
Machine vision systems have made significant strides in animal sciences, particularly in tasks such as animal counting. These systems offer substantial benefits in terms of time-saving and enhancing husbandry practices. The process of teaching machine vision systems to count animals is based on clear knowledge structures developed by human intelligence. For example, we humans know how to count animals. We can, for example, establish a reference point, such as a gate, and then count each time an animal crosses that reference point. Machine vision systems can be trained using this principle by programming them to identify specific features or characteristics of animals and then applying algorithms to detect when this recognized object (animal) passes through the designated reference point.
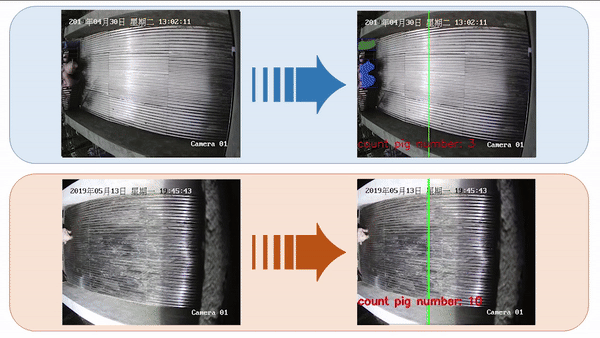
Video: Automated pig counting using machine learning. Source: Pigs on conveyor belt; kaggle.com
These machine vision systems are built upon our understanding of counting principles, which are then translated into algorithms and training data. Such counting methods or similar spatial location AI systems simply mimic human capabilities. However, AI systems without clear knowledge structures simply don’t work. It is not uncommon to see companies or researchers showing “demos” that use machine vision or other AI methods and showing how these “disruptive technologies” will change the animal sciences. However, in most cases, these technologies are primarily used for descriptive purposes and lack predictive power. If these “AI demos” do not offer any predictive power or if these models do not have clearly defined knowledge structures, they are merely showcasing speculative value. Tech companies often rely on speculative value to increase their worth and attract funding to sustain high salaries and other expenditures. Nevertheless, this approach can result in future losses for their investors if the technology fails to produce tangible results. Thus, it’s essential to consider that “AI demos” can and are being used as a marketing strategy. We need to exercise caution and gain a clear understanding of what AI can and cannot achieve in the field of animal sciences. This understanding will help us allocate our resources to develop effective problem-solving strategies.
AI won’t take your job
As previously discussed, AI relies heavily on human intelligence; without it, these systems would be rendered useless. In the realm of animal science, numerous challenges persist, such as defining and measuring optimal animal welfare, assessing optimal health, and improving various processes in animal production. These endeavors continue to rely on human cognition to navigate the complexities of such dynamic systems. While there are a few practical applications where AI shows promise, such as pig counting or detecting signs of specific diseases, these applications are expected to yield only marginal improvements compared to the vast array of factors influencing livestock production. As a result, they are far from reaching a stage where they require minimal human intervention. Thus, unless your role is specifically centered around pig counting, your job is not at risk. As an industry, we rely on human intellect to conduct the fundamental research necessary to develop the knowledge structures that may lead to practical AI systems in the future.
Thanks for reading!
Christian Ramirez-Camba
PhD in Animal Science
MS in Data Science
*Please note that the opinions expressed in this blog post represent only the authors’ views and do not reflect those of the Animal Science Data Lab, the associated institutions, or their sponsors